
Science in the Age of AI: Can We Trust Gen AI in Health Tech?
Science in the Age of AI: Can We Trust Gen AI in Health Tech?
A Neuroscientist’s Guide to Using Gen AI in Health Tech
As a neuroscientist and business owner with a lifelong curiosity about human behaviour, I’ve spent a lot of time exploring how these tools work, both in academia and business settings. And the contrast between how scientists and non-scientists use Gen AI is fascinating.
This blog isn’t here to tell you AI is bad. When used thoughtfully, it can be incredibly helpful. But if you’re working in health tech, there are real risks to be conscious of. Using Gen AI without being aware of these risks can affect the accuracy of your product, the credibility of your brand, or even the safety of your users. Instead, I’m going to tell you when you can use AI, when you shouldn’t, and why.
In this guide, I’ll walk you through:
- how scientists are currently using AI in their work (and what they’re worried about)
- the most common ways businesses are using these tools and where things tend to go wrong
- how to use AI tools to automate scientific tasks in your business
In another post, I review the best AI tools for research and how to use them.
How Scientists Use Gen AI in Research
If you’re a business using AI tools like ChatGPT, Claude or Perplexity in your workflows, it’s worth knowing how scientists are actually using these tools and (just as importantly), how they aren’t. So how is AI used in science?
A recent preprint study from a group including the University of Washington, Princeton, and the Allen Institute surveyed 816 verified academic researchers about how they use large language models (LLMs).
They found that over 80% of researchers were already using tools like ChatGPT in their research workflows. But most of that use was tightly focused on specific, repetitive and low-risk tasks. Scientists rely on these models to support their thinking, not replace it.
Some of the most common uses include:
- Information seeking: searching for papers, generating summaries, asking broad field-level questions
- Editing: rewriting text, improving clarity, formatting, and translating ideas into fluent English
- Ideation: brainstorming new research questions or ways to frame a problem
- Data cleaning or analysis (in specific computational tasks)
- Rewriting, shortening or summarising content directly
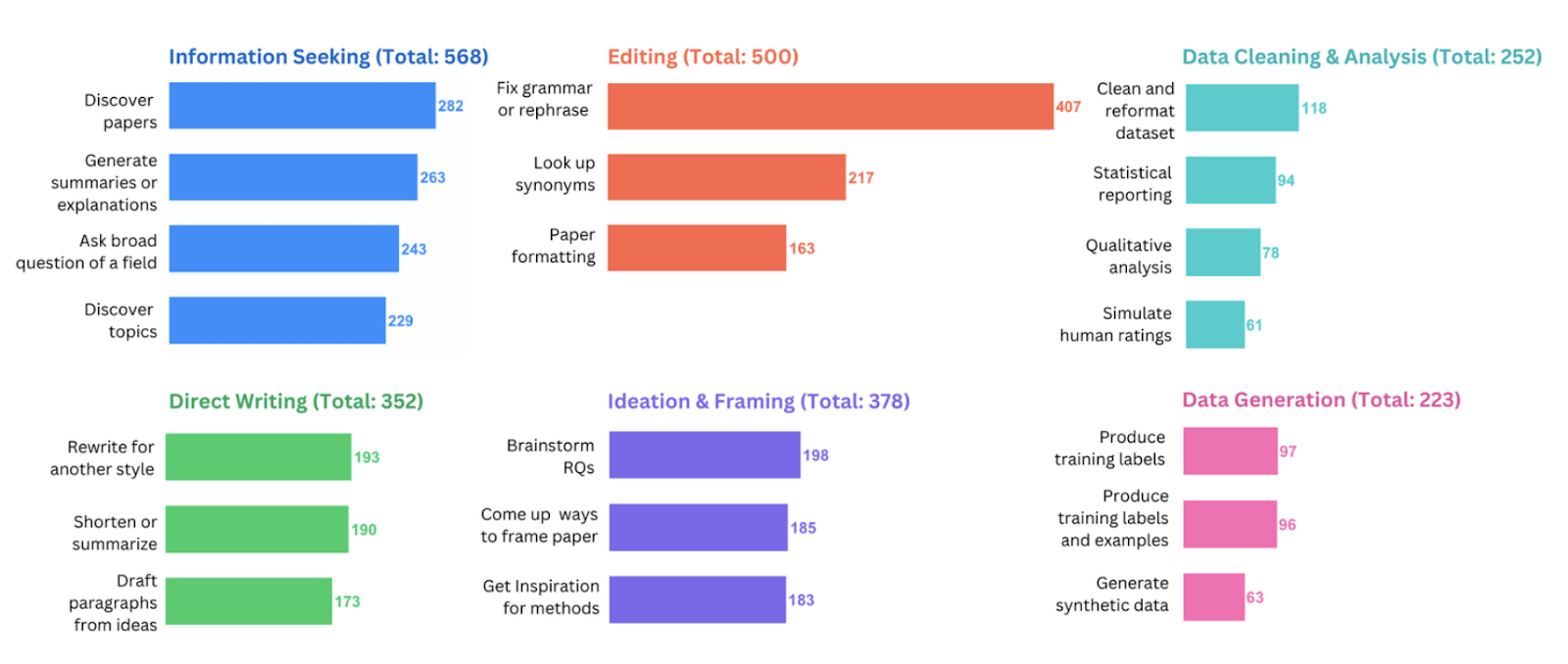
Critically, researchers don’t treat AI as a source of truth. They use it as a tool to speed up familiar tasks, like things they already know how to do but want to do faster or more efficiently.
And this makes a big difference.
Because researchers have deep expertise, they know what to ignore. When AI suggests a fringe paper from a low-quality journal, or includes outdated data, they’re more likely to spot it. They’ve spent years learning how to read between the lines — scanning methods for bias, checking citations, knowing when a study’s results don’t match its conclusions. They also often have institutional access to all the literature, not just the ~30% that’s open access and visible to AI tools.
That’s a crucial caveat. Just because scientists are using AI to search for information or generate ideas doesn’t mean this is a safe use for non-experts. Those without a scientific background might not have the context to spot red flags, which increases the risk of being misled. Or worse, building products and making claims based on flawed information.
Which scientists are using Gen AI?
What’s interesting is which scientists are using Gen AI and why. And how does this relate to the world of health tech?
Interestingly, it’s not the most senior scientists leading AI adoption, it’s often those with the most to gain.
The same study found that junior researchers, non-native English speakers, and scientists from marginalised backgrounds were more likely to use GenAI, especially for tasks like writing and publishing. Meanwhile, senior researchers, women, and non-binary scientists reported more ethical concerns and were less likely to adopt AI tools.
A separate Nature Human Behaviour study found that using AI-related terms in publications boosts citations, but that boost isn’t equal. Underrepresented groups weren’t cited as much, even when using the same tools, suggesting that AI could deepen existing inequalities in science.
It’s worth reflecting on how this maps to the business world. We already know that women and non-binary founders already receive less VC funding. If those same groups are also less likely to use AI, or they aren’t being offered the same education and training to use it responsibly, there’s a real risk that generative AI could amplify existing disparities rather than reduce them.
The red flags scientists are seeing in AI
If researchers are embracing AI, what’s the catch? What does the emergence of ChatGPT and Generative AI mean for science?
Despite the excitement around AI’s potential in science, scientists are also some of the most vocal about its risks. According to a 2025 survey of nearly 5,000 researchers in more than 70 countries, the things researchers are most concerned about are ethical concerns, followed by inaccuracies and hallucinations.
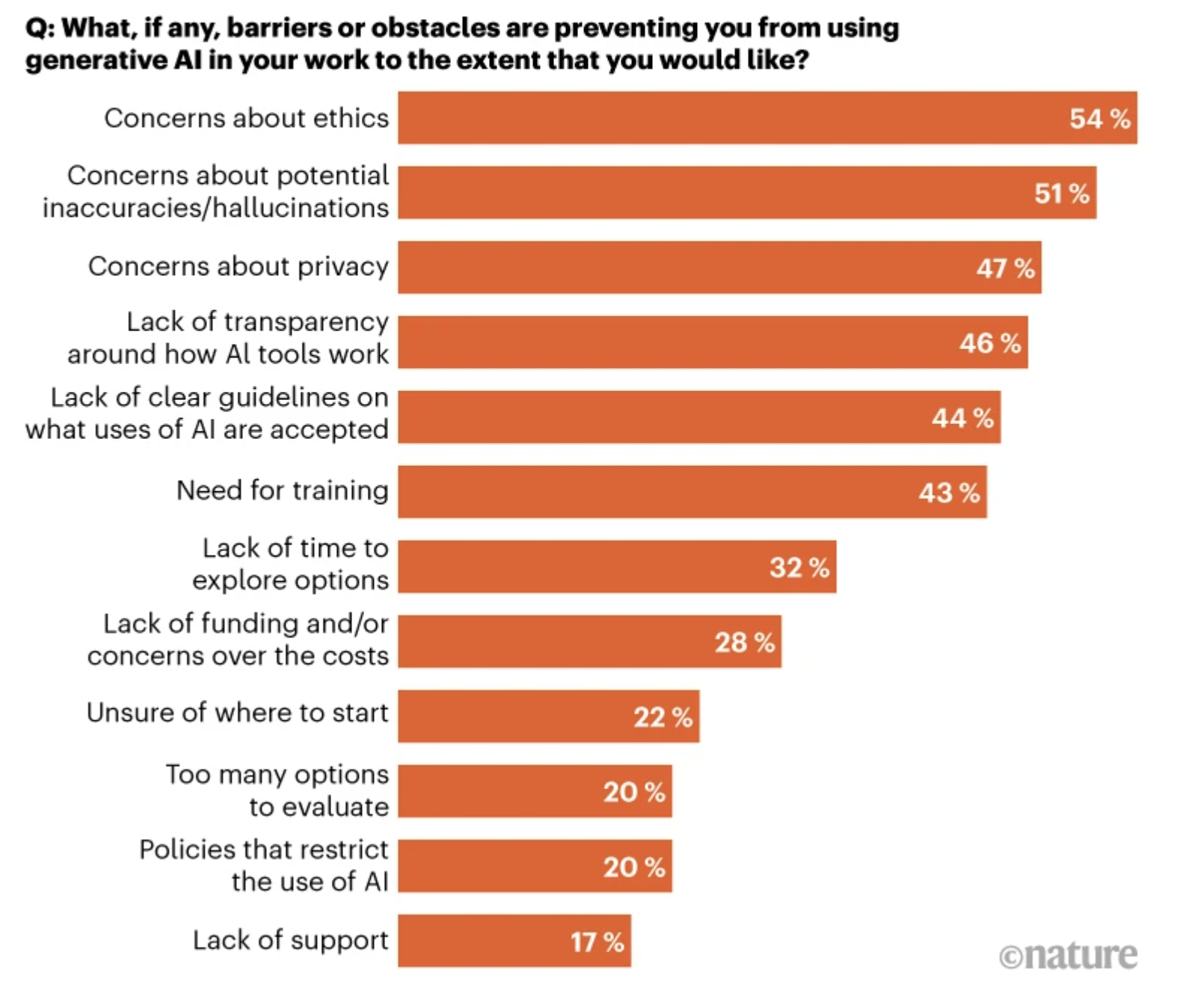
Beyond technical errors, AI introduces a new kind of risk to the integrity and direction of science itself. It can change how we create knowledge in ways that are invisible and possibly damaging.
A 2024 paper from researchers at Yale and Princeton coined a powerful phrase for this:
“The illusion of understanding.”
The authors argued that relying too heavily on AI in these roles can distort how knowledge is generated, especially if AI shapes what questions we ask, which methods we use, and what kinds of studies get prioritised. The result is what they call a monoculture of knowing.
“There is a risk that scientists will use AI to produce more while understanding less.” — Lisa Messeri
A monoculture of knowing means:
- favouring fast, measurable, AI-compatible questions
- neglecting slower, more complex modes of inquiry (like patient interviews, ethnographic studies, or behavioural observations)
- mistakenly believing that AI is objective, when in fact it reflects the worldview of the people who built it
Academic institutions are beginning to respond. The US National Institutes of Health (NIH) has issued formal guidance against using AI tools to write grant applications or in the peer-review process, citing concerns around originality of thought, intellectual diversity, and even research misconduct.
So, what does this mean for businesses?
If you're using AI to guide how you design your product, frame your research, or communicate with users, it's vital to understand that these tools are not neutral. They reflect patterns in existing data and that data might not represent your audience, your goals, or the nuances that matter in healthcare. Worse, they might appear to do so, giving you a false sense of security that you’re covering all bases.
The risk isn’t just technical. It’s philosophical. If you only ask questions that AI is good at answering, you may miss the questions that actually matter.
Innovation in health tech comes not from mimicking what’s already been done, but from paying attention to complexity, diversity, and lived experience. And that’s something no model can fully replicate. This doesn’t mean you shouldn’t use AI, it means you should know when to use AI.
How startups are using AI to interpret science
For startups, the appeal of AI is obvious: instant answers, fast content, and a confident tone. All at a fraction of the time or cost of hiring a domain expert.
Indeed, in his new book The Experimentation Machine, Jeffrey J. Bussgang says,
“Startup founders who use AI are going to replace founders who don’t.”
But if scientists are already warning about over-reliance on AI, what does that mean for businesses using the same tools to guide product decisions, interpret clinical evidence, or draft investor updates?
From what I’ve seen on LinkedIn, news articles, literature reviews, and research studies, here’s how most startup teams are using Gen AI tools like ChatGPT, Claude, or Perplexity in their day-to-day work:
- Generating blog posts and marketing copy that reference studies
- Summarising academic research papers
- Explaining complex scientific terms
- Drafting grant applications or pitch decks
- Simulating user journeys or health personas
- Researching product–market fit potential
In some cases these uses can be helpful, particularly when time is short and you're just trying to get your head around a new concept. But in health tech, where scientific credibility and accuracy matter, the risks are much greater than they first appear.
Challenges of Using AI in Healthtech
Gen AI use becomes particularly risky in health, where decisions are often based on subtle differences in methodology, participant demographics, or clinical relevance (things most AI tools are not trained to evaluate). This matters because health is messy. People are complex. And AI, by design, tends to oversimplify. Here are two of the most common challenges of Gen AI in health tech:
False confidence and hallucinations
AI models are often most dangerous when they’re wrong with confidence. The outputs are well-written, often come with references, and seem trustworthy at first glance. But that surface-level polish can be dangerously misleading.
I’ve seen this firsthand. Clients have sent me grant applications drafted with ChatGPT that included references to podcasts (which would lead to an automatic rejection from any serious funding body). In other cases, the citation looked entirely plausible until I checked the actual paper, only to find it had nothing to do with the claim they were making.
The problem is if something looks correct at first glance, your brain often relaxes. It’s cognitively harder to stop and engage in critical thinking, especially when you’re under time pressure. Indeed, a new McKinsey report shows that 30% of companies will only check a small proportion of their gen-AI-produced content before use, while only 27% of companies will thoroughly review all its outputs.

Unfortunately, companies that use AI-generated content for grant applications, blog posts, or investor decks may unknowingly include hallucinated studies or misattributed references, all delivered in a polished, persuasive tone.
A 2023 study from Vectara found that some chatbots hallucinate up to 27% of the time. Another BBC report found that nearly 50% of AI-generated responses to news-related queries contained factual errors. Or as Steve Blank, a Professor at Stanford University, puts it,
“Somewhere between 10% and 50% of their answers are bullshit, sure. And the problem is you don’t know which ones.”
You won’t get a warning when something’s off. And unless you have a trained scientist reviewing the output, you may not realise it until someone calls it out.
Simulating user perspectives lacks nuance
Some startups are starting to use AI-generated synthetic users to simulate feedback, test user journeys, or even do early market research. While this can seem efficient (why talk to a customer when you can simulate one?), it comes with serious limitations.
Take mental health, for example. If you ask ChatGPT to describe a patient with depression, you’ll probably get a clean, textbook-like description of melancholic depression, a well-defined subtype that aligns neatly with many diagnostic criteria. But that’s only one version of the condition. Other subtypes, like atypical depression, are common in clinical settings with some studies suggesting they account for up to 40% of cases.
The problem is that atypical depression looks almost like the opposite of melancholic depression. Where melancholic patients often experience early-morning waking, loss of appetite, and emotional numbness, those with atypical depression may sleep excessively, crave carbohydrate-rich foods, and display emotional reactivity. These distinctions matter, especially when designing products or behaviour-change interventions.
A human scientist would flag that and conduct representative research. An AI won’t.
AI-generated responses are based on averages and surface-level traits, not lived experience. They can flatten real diversity into neat categories and fail to reflect the emotional, cultural, or behavioural complexity of actual people. Relying on synthetic data can give you a false sense of insight and lead to products that exclude marginalised groups, misrepresent your evidence, and lead to poor outcomes.
If you’re serious about designing for health, synthetic empathy isn’t enough.
What does this mean for your startup?
It doesn’t mean you shouldn’t use AI. But it does mean that health tech startup teams need a different playbook than SaaS startups using AI to write marketing emails.
In health tech, credibility can make or break your product. Certain tasks that require scientific insight, ethical nuance, and research integrity still need an augmented approach with expert oversight. You can use AI to accelerate parts of the process, but create guidelines and use-cases so that your team know when and how to use it.

AI tools for scientific research: Should you use them?
Sam Altman, CEO of OpenAI, says he only uses AI for “boring tasks” — things like summarising documents or drafting emails. Not for critical thinking. Not for making decisions that carry real risk (unless you create a bespoke model that you train yourself). And that’s a good heuristic for startups and companies too.
For other tasks, you can use a simple rule of thumb: If you wouldn’t feel confident defending the science yourself, don’t outsource it to AI.
Instead, what you should do is follow these practices to leverage AI responsibly:
- Augment, don’t replace: Use AI for iterative tasks (like summarising, formatting, or data cleaning), but keep humans in the loop for all critical thinking, interpretation, and decision-making.
- Bias mitigation: Actively audit AI outputs, especially when your product addresses underserved or marginalised populations. AI reflects its training data, and that data may not include the people you serve.
- Validation protocols: Set strict review processes for any AI-generated scientific content. If you're in a regulated space, this isn't optional, it's a liability if you don’t.
- Expert collaboration: Pair AI tools with scientists, clinicians, or researchers who can spot gaps and keep your work grounded in actual evidence.
- Train bespoke models: Instead of relying on publicly available Gen AI tools, integrate bespoke models trained on a limited set of verified data into your company's workflows to ensure reliability and compliance.
For a review of which publicly available Gen AI tools you should use, see my other post evaluating the best AI tools for research.
What AI means for health tech
With Generative AI, anyone can ask complex questions, generate summaries, and access research instantly (no PhD or institutional login required).
But that accessibility comes with a risk: a false sense of understanding. When AI outputs are clean, confident, and well-structured, it’s easy to assume they’re also correct. In health tech, that assumption can lead to real harm. AI can help you find information, but it still can’t interpret it. It won’t ask if the study was biased, whether the right question was posed, or which patient groups were left out.
A recent framework published in PNAS outlines four key factors that determine whether a scientific task can be automated:
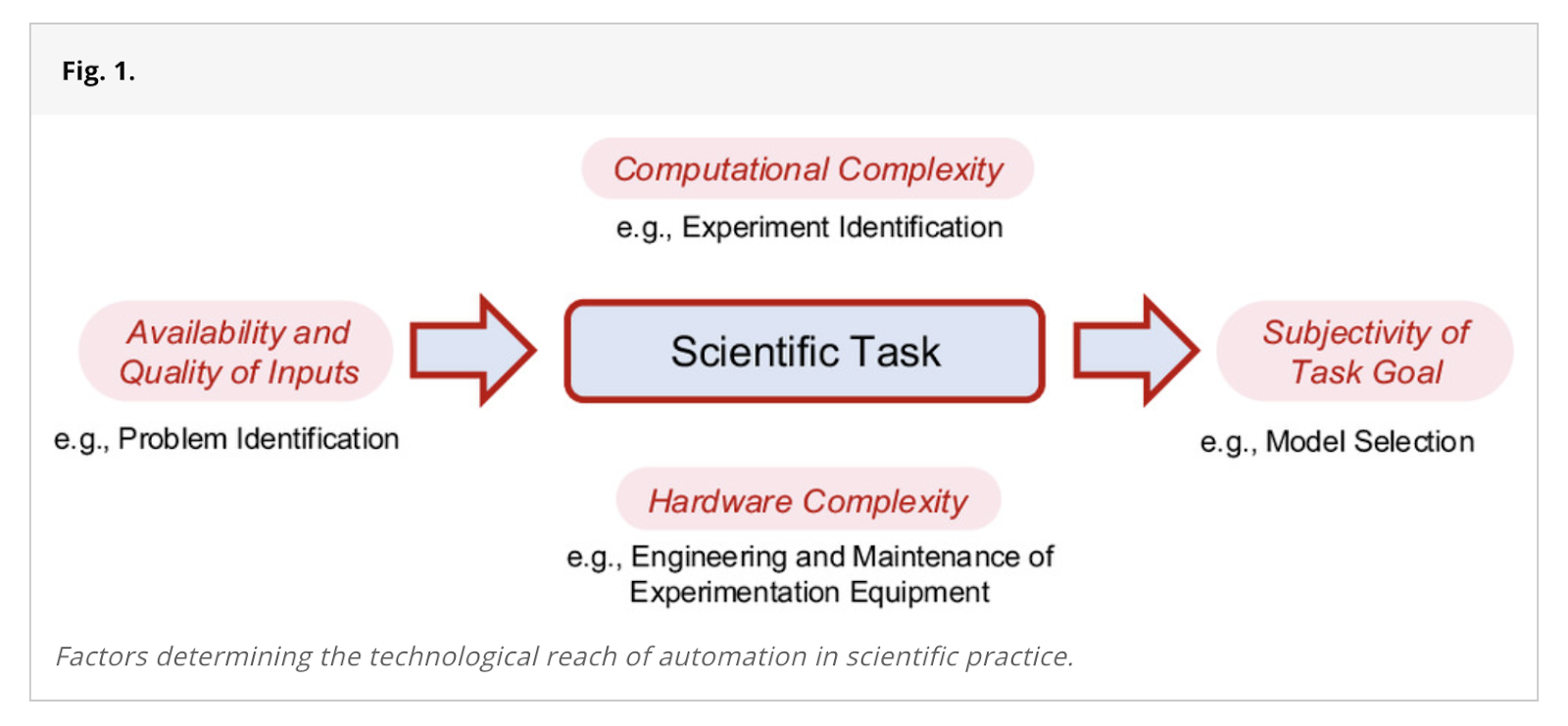
Evaluating the complexity of these factors helps to define the technological boundaries of what AI can realistically automate in scientific work. The authors also reinforce the idea that complete automation isn’t desirable or feasible.
In health tech, many critical tasks are deeply contextual and often subjective (like formulating research questions, selecting the right model, or designing a clinical study). These are exactly the areas where AI struggles most. That’s why a human (scientist) insight is still essential.
At Sci-translate, we combine rigorous scientific thinking with real-world knowledge. We know when and how to use AI tools effectively. We also know where human judgement is essential to ensure accuracy, relevance, and responsible innovation.
Get Expert Support
If you're building in health tech or want to use AI responsibly without compromising on credibility, our team of experts can help.
At Sci-translate, we offer:
Book a call with me (Dr Anna McLaughlin, founder of Sci-translate), to get started.
Let’s make sure your science stays sound, even in the age of AI.